Four Spatial Analysis Techniques to Boost Outcomes in 2017
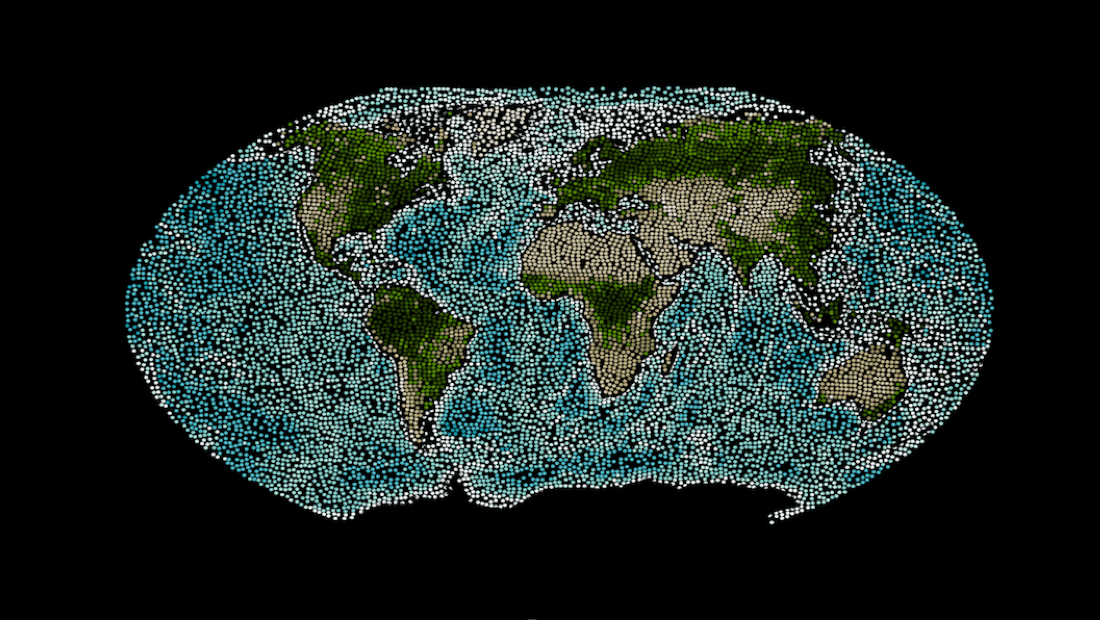
The debut of Apple’s iPhone in 2007 ushered in a location data revolution. The exponential rise in mobile devices crowdsourcing applications and smart gadgets connected within the Internet of Things over the last decade however has generated an unprecedented amount of location data leading to what some are calling Location Data 2.0.
The ability to transform location data into business outcomes has become a new litmus test within our data-driven economy and companies are employing Location Intelligence tools like spatial analysis to pass this test while also surpassing the competition.
Javier de la Torre our CEO recently hosted a webinar examining the new role of location data and its impact within the business community.
The availability of and access to location data Javier explained has alerted businesses to new optimization strategies and has altered the foundation upon which businesses are modelled. Uber for instance could not offer Uber Pool without customer location data.
During the webinar Javier highlighted four types of spatial analysis expected to gain popularity in 2017.Let’s take a look at how each technique can transform location data into business outcomes.
1. Spatial Optimization for Dynamic Routing
A driving force behind many data implementation initiatives is the promise of operational “optimization.” This dimension is constantly on the move which has caused problems for analysts measuring its success.
Businesses applying spatial optimization to location data however are relocating the meaning of “optimization.”
Waste management companies for instance are benefiting from the abundance of available location data especially within smart cities in an attempt to optimize resource allocation.
Whether managing fleet deployment or collection frequency spatial optimization can be applied to location data such as waste volume measurements along routes to determine collection frequency.
2. Linear Programming for Constrained Optimization
Waste management optimization also requires taking into account city-specific constraints which can be accomplished with Linear programming.
This spatial analysis technique accounts for contextual conditions to determine the optimum outcomes given site-specific constraints.
Returning to the example of waste management managers can use linear programming to create route assignments that reduce collection frequency and by extension fuel costs.
At the same time linear programming factors into the equation external constraints such as waste disposal facilities. In order to reduce collection frequency waste collection services must ensure that disposal facilities are not overloaded on any given day.
Did you miss the webinar? Watch it anytime on-demand! Download
3. Gradient Boosting Models and Machine Learning
The latest turn in the location data revolution is shifting toward gradient boosting and machine learning to generate optimization algorithms for future price predictions.
In March 2017 Foursquare’s CEO Jeff Glueck introduced Foursquare Analytics a dashboard promising to put the company’s proven power of “location intelligence in the hands of brands.”
Aspiring to emulate the surprising success of retailer [T. J. Maxx] Foursquare Analytics measures “chain-level foot-traffic performance.”
In the clip below Javier discusses how the business model of Foursquare Analytics is predicated upon large volumes of location data provided from user “check-ins.”
More specifically Javier examines Foursquare’s analysis of fast-food chain Chipotle following last year’s E. coli contamination outbreak and discusses the difference in forming predictions based on sales data versus in-store foot-traffic. Take a look:
Although the year-over-year decline in sales showed little changes in-store customer foot-traffic showed signs of improvement in Q1 of 2017.
As such location data seems to offer a more robust dimension for both measuring chain-level performance as well as predicting future trends and patterns.
4. Indoor Analytics
That Foursquare turned toward location intelligence after studying T. J. Maxx’s success is not necessarily surprising given that retailers have been increasing revenue for some time with location intelligence.
Indoor analytics like machine learning build gradient boosting models with location data not only to predict in-store traffic more accurately but also to understand customer segments and behavioral patterns more clearly.
Indoor analytics can reduce overhead spending as data visualizations featuring custom basemaps for individual stores can spotlight service gaps in high-traffic areas requiring a reallocation of staff resources.
Conclusion
June 29 2017 will mark the ten year anniversary of the iPhone and the location data revolution more generally.
The business landscape has changed in the intervening ten years and will continue to do so as more industries start transforming location data into business outcomes through the tools and techniques of Location Intelligence.
Uber and Foursquare are cornering a market predicated on leveraging and licensing location data and expect further changes in 2017 to traditional industries ranging from waste management retail and even fast-food.
Whether applying spatial analysis to location data to improve optimization or prediction depends upon the “where factor ” and Location Intelligence provides the wherewithal to businesses joining the revolution.
Happy Data Mapping!